Winning with numbers: Understanding Sports Data Analytics Simplified

Understanding Sports Data Analytics
Sports enthusiasts have always enjoyed analyzing and discussing their favourite teams and players. With the rise of data analytics in sports, fans now have access to an abundance of information that can help them gain a deeper understanding of the game. From player performance to team strategy, sports data analytics has become an integral part of the game. However, for most people, the world of sports analytics can be overwhelming and intimidating.
In this post, we will simplify the world of sports data analytics, breaking down complex concepts into easy-to-understand terms. We will explore how sports data analytics works, its benefits, and how to use it to your advantage. Join us as we explore the exciting world of sports analytics and discover how you can use it to win big!
Introduction to sports data analytics
Sports data analytics involves the application of data analysis techniques to sports-related data in order to gain insights and make informed decisions. Here’s a simplified overview of sports data analytics:
- Data Collection: Relevant data is collected from various sources such as game statistics, player performance metrics, match footage, sensor-based tracking systems, and social media.
- Data Cleaning and Preparation: The collected data is processed and cleaned to remove errors, outliers, and inconsistencies. It is then organized and formatted for analysis.
- Data Exploration: Analysts explore the data to identify patterns, trends, and relationships. They may use visualizations, statistical techniques, and exploratory data analysis to gain a better understanding of the data.
- Performance Analysis: Data analytics is used to evaluate player performance, team performance, and tactical strategies. Metrics such as goals scored, assists, shooting accuracy, pass completion rates, and defensive actions are analyzed to assess player and team effectiveness.
- Player Evaluation and Scouting: Data analytics helps in evaluating individual players and identifying talent. Metrics such as player ratings, contribution to team success, and potential indicators are analyzed to assess player performance and potential.
- Opponent Analysis: Analytics is used to analyze opponents’ strengths and weaknesses, playing styles, and strategies. This information helps teams develop game plans and make tactical decisions to gain a competitive advantage.
- Injury Prevention and Player Health: Data analytics is utilized to monitor player workload, injury risk, and recovery patterns. It helps in identifying potential injury risks, optimizing training programs, and making data-driven decisions to prevent injuries and maintain player health.
- Fan Engagement and Experience: Data analytics is used to understand fan preferences, behaviour, and sentiment. It helps teams and organizations deliver personalized experiences, optimize ticketing strategies, and improve fan engagement through targeted marketing campaigns.
- Decision Making and Strategy: Insights gained from data analytics assist coaches, managers, and executives in making data-driven decisions. This includes selecting lineups, making substitutions, designing training programs, formulating game strategies, and evaluating team performance.
- Predictive Analytics: Advanced techniques such as machine learning and predictive modelling are applied to predict game outcomes, player performance, and other sports-related events. These predictions can help in decision-making and strategy development.
Sports data analytics simplifies the process of analyzing sports-related data to extract valuable insights. It enables teams, coaches, and organizations to optimize performance, make informed decisions, and improve overall outcomes in the competitive world of sports.
You can also see Top 10 Most Watched Sporting Events in USA
The basics of sports data analytics
Sports data analytics is a powerful tool that is widely used in the world of sports. Whether you are a coach, team owner, or player, understanding the basics of sports data analytics can give you a competitive edge. At its core, sports data analytics involves the collection, analysis, and interpretation of data to help teams make informed decisions about everything from player recruitment to game strategy.
One of the key components of sports data analytics is the use of statistical analysis. This involves taking data from various sources, such as player performance stats, game statistics, and even weather conditions, and using advanced mathematical techniques to identify patterns and trends. This can help teams to identify their strengths and weaknesses, as well as those of their opponents, and to develop strategies to overcome them.
Another important aspect of sports data analytics is the use of predictive modeling. This involves using historical data to make predictions about future events. For example, by analyzing data on player performance, you can make informed predictions about how a particular player might perform in a given game or season. This can help teams to make more informed decisions about player recruitment, as well as their game strategy.
Overall, sports data analytics is a powerful tool that can provide valuable insights into the world of sports. By understanding the basics of sports data analytics, you can gain a competitive edge and take your team to the next level.
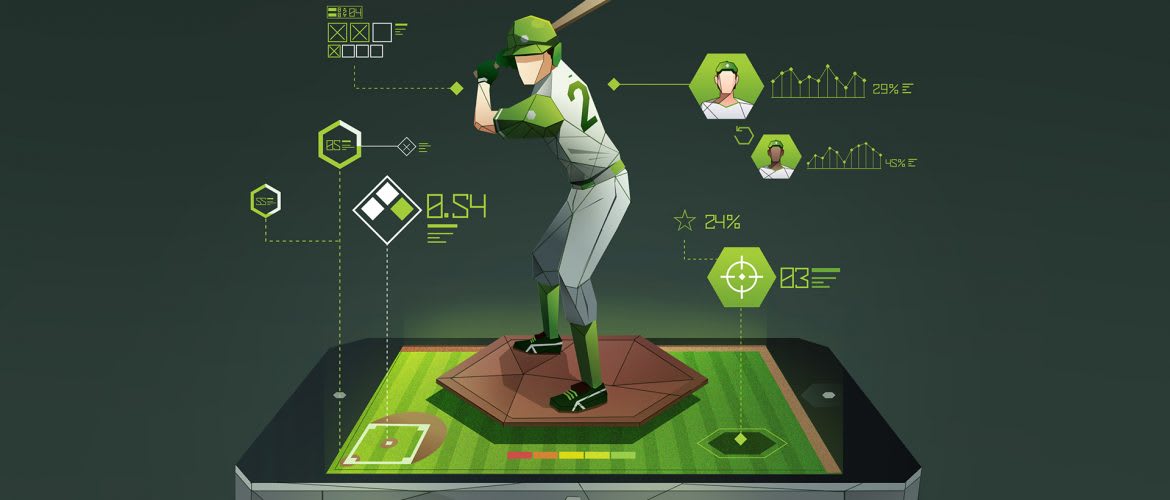
The importance of data in sports
Data analytics has become an integral part of sports in recent years. The importance of data in sports cannot be stressed enough, as it provides valuable insights into player performance, team strategy, and game outcomes. Sports organizations across the world are investing heavily in data analytics to gain a competitive edge, and it’s not difficult to see why.
Data analytics in sports involves collecting data from various sources, including video footage, sensors, wearables, and other devices, to provide a comprehensive picture of player and team performance. This data is then analyzed using sophisticated algorithms to identify patterns, trends, and insights that can be used to make informed decisions about player selection, training, and game strategy.
The use of data analytics in sports has transformed the way teams are managed and games are played. Coaches can now make data-driven decisions, based on real-time insights, rather than relying on gut instincts or past experiences. They can identify areas of weakness in their team and opponents, and adjust their game strategy accordingly. Players can also benefit from data analytics, as it provides them with valuable feedback on their performance, helping them to improve their game.
Overall, the importance of data in sports cannot be overstated. It has become a game-changer, providing valuable insights and helping teams to achieve better results on the field. With the growing popularity of data analytics in sports, we can expect to see even more exciting developments in the future, as teams continue to leverage data to gain a competitive edge.
Common sports data metrics and terminology
When it comes to sports data analytics, there are a few common metrics and terminology that you need to be familiar with. These metrics and terminology will help you understand the data you are analyzing and how it relates to the performance of the team or player in question.
One of the most common metrics is “win-loss record”. This metric simply refers to the number of games a team or player has won versus the number of games they have lost. This metric is used to gauge the success of a team or player over a period of time.
Another important metric is “points per game”. This metric is commonly used in sports like basketball, football, and hockey to measure the scoring ability of a team or player. It is calculated by dividing the total number of points scored by the number of games played.
“Shots on goal” is another important metric used in sports like soccer and hockey. This metric measures the number of times a team or player has taken a shot at the goal during a game.
Other common sports data terminology includes “assists”, which refers to the number of times a player has helped another player score a goal or make a play, and “rebounds”, which refers to the number of times a player has grabbed a missed shot in basketball.
Understanding these common sports data metrics and terminology will give you a solid foundation for analyzing and interpreting sports data analytics. By using these metrics in your analysis, you can gain insights into the performance of teams and players, and make informed decisions based on the data.
The role of analytics in decision-making for sports teams and organizations
Analytics play a crucial role in decision-making for sports teams and organizations. In the past, decisions were often made based on gut feelings or personal biases. However, with the advancements in technology and data analytics, it’s now possible to make informed decisions based on real data and insights.
Sports organizations now have access to vast amounts of data, ranging from player performance stats to fan engagement metrics. By analyzing this data, teams can identify patterns and trends that can help them make better decisions about player recruitment, game tactics, and even marketing strategies.
For instance, data analytics can help teams to identify the strengths and weaknesses of their players and opponents. This knowledge can be used to develop game plans that capitalize on strengths and exploit weaknesses, ultimately increasing the chances of winning.
In addition, data analytics can be used to improve player performance and prevent injuries. By monitoring player data, teams can identify when players are at risk of injury and take preventive measures to keep them healthy.
Overall, analytics have become an essential tool for sports teams and organizations looking to gain a competitive edge in their respective leagues. By leveraging data insights and making informed decisions, sports organizations can increase their chances of winning and achieve greater success both on and off the field.
10 Scientific Benefits of Playing Sports Everyday
Examples of successful applications of sports data analytics
Sports data analytics has been leveraged by many successful teams and organizations to gain a competitive advantage. Here are some examples of how sports data analytics has been successfully applied in the sports industry:
1. The Golden State Warriors, a professional basketball team, used data analytics to evaluate players’ shot selection and find more efficient ways to score. By analyzing shooting patterns, the Warriors were able to identify that their players were more successful shooting from certain areas of the court, and they used this information to adjust their game strategy.
2. The Seattle Seahawks, a professional football team, used data analytics to optimize their game strategy. They analyzed player performance data to identify which plays were most successful, and used this information to develop a game plan that was tailored to their strengths.
3. The Houston Astros, a professional baseball team, used data analytics to identify undervalued players and build a winning team. By analyzing data on player performance, the Astros were able to identify players who were overlooked by other teams but had the potential to be valuable contributors.
4. The Leicester City football club, a professional soccer team, used data analytics to guide their recruitment strategy. They analyzed data on player performance and identified undervalued players who could help them achieve their goals. This approach proved to be highly successful, as Leicester City went on to win the English Premier League in 2016.
These examples demonstrate the power of sports data analytics to transform the way teams operate and achieve success. By leveraging data to gain insights into player performance, game strategy, and recruitment, teams can gain a competitive advantage and achieve their goals.
Top 10 Latest Sports Technology
Key challenges in sports data analytics
Sports data analytics has brought a new dimension to the world of sports, but it’s not without its challenges. Gathering and analyzing data is a complex process that requires a lot of time and resources. Some of the key challenges in sports data analytics include data quality, privacy concerns, and the interpretation of results.
One of the biggest challenges is ensuring the data is accurate, complete, and up-to-date. Data quality is essential to the success of any analysis, but it’s particularly important in sports. Sports data is often manually collected, which can lead to errors and inconsistencies. It’s critical to have a system in place to validate and verify the accuracy of the data.
Privacy concerns are also a significant challenge in the world of sports data analytics. Athletes and sports organizations are understandably protective of their data, and there are strict regulations governing how it can be used. It’s important to ensure that all data is collected and analyzed in compliance with relevant privacy laws and regulations.
Finally, interpreting the results of sports data analysis can be challenging. There is a vast amount of data available, and it can be challenging to know how to interpret it effectively. Understanding the context in which the data was collected and being able to draw meaningful insights and conclusions is critical to the success of any analysis.
Despite these challenges, sports data analytics has the potential to revolutionize the world of sports. By gaining a better understanding of the data, teams can make more informed decisions, improve performance, and gain a competitive edge.
Top 10 Biggest Sportswear Brands in the World
Limitations of sports data analytics
Sports data analytics has revolutionized the way we understand sports. It has opened up new avenues for teams, players, and fans alike. However, as with any technology, there are limitations to what sports data analytics can achieve.
One of the key limitations is the accuracy of data. Although technology has improved significantly over the years, there is still some degree of error in the data collected. For instance, in baseball, the accuracy of pitch tracking systems may be compromised due to the occurrence of spin rate changes, which can be affected by environmental conditions as well as player fatigue.
Another limitation is the use of data in isolation. Data analytics provides insights based on data, but it is important to understand that data alone cannot give a complete picture of a team’s performance. There are many factors that can influence a team’s performance, such as injuries, team dynamics, and coaching strategies.
Finally, there is a danger of overreliance on data analytics. As with any tool, it is important to use sports data analytics appropriately. There is a danger that coaches and teams may become over-reliant on data analytics, and fail to consider other important factors such as intuition and experience.
In summary, sports data analytics is a powerful tool that has revolutionized sports. However, it is important to understand its limitations and use it appropriately to gain the best insights possible.
10 Lesser Known Facts about Ryan Garcia
Ethical considerations in sports data analytics
As with any form of data analytics, ethical considerations are paramount in sports data analytics. The use of data analytics in sports has increased in recent years and it has revolutionized the way teams prepare and compete. However, it is important that teams and organizations using such methods are ethical in their practices.
One ethical consideration is the use of personal player data. It is important that player data is collected and used in a way that is respectful of players’ rights and privacy. This means that teams should only collect data that is necessary and relevant for the purposes of improving performance and not use it for any other purpose without the player’s consent.
It is also important that teams do not use data analytics to discriminate against players based on factors such as race, gender, or other personal characteristics. This means that teams should ensure that data analytics is used in a fair and unbiased manner.
Another ethical consideration is the use of data analytics in sports betting. While sports betting is legal in some jurisdictions, it is important that teams and organizations do not use data analytics to influence the outcomes of games or to gain an unfair advantage in sports betting.
In conclusion, ethical considerations are an important aspect of sports data analytics. Teams and organizations using such methods should ensure that they are ethical in their practices and ensure that they are collecting and using data in a way that is respectful of players’ rights and privacy. By doing so, they can use data analytics to improve performance and compete at the highest level while maintaining ethical standards.
Extreme adventure vacations in the USA to try before you die
Future trends and developments in sports data analytics
Sports data analytics has come a long way in the past few years, but the future is even more exciting. The use of machine learning and artificial intelligence in sports data analytics is expected to grow rapidly. These technologies will allow for more accurate predictions and insights into player performance, injury risks, and more.
One area where machine learning and AI are expected to make a big impact is in player recruitment. By analyzing a player’s performance history, teams can identify players who are likely to perform well in their system. This can help teams make more informed decisions when it comes to signing new players.
Another trend to watch in sports data analytics is the use of wearable technology. Wearables, such as fitness trackers and smartwatches, are already being used by athletes to track their performance and health. In the future, we can expect to see more sophisticated wearables that are designed specifically for sports performance. These devices will allow teams to collect even more data on their players and make more informed decisions.
Finally, we can expect to see more collaboration between sports teams and data analytics companies. As more teams begin to realize the benefits of data analytics, they will need help analyzing and interpreting the data. This presents an opportunity for data analytics companies to work with teams to develop customized solutions that meet their specific needs.
Overall, the future of sports data analytics is bright, and we can expect to see continued growth and innovation in this field in the years to come.
You can also see Mobile Games Coming Soon to Netflix in 2023